AlphaDDA: strategies for adjusting the playing strength of a fully
Por um escritor misterioso
Last updated 31 dezembro 2024
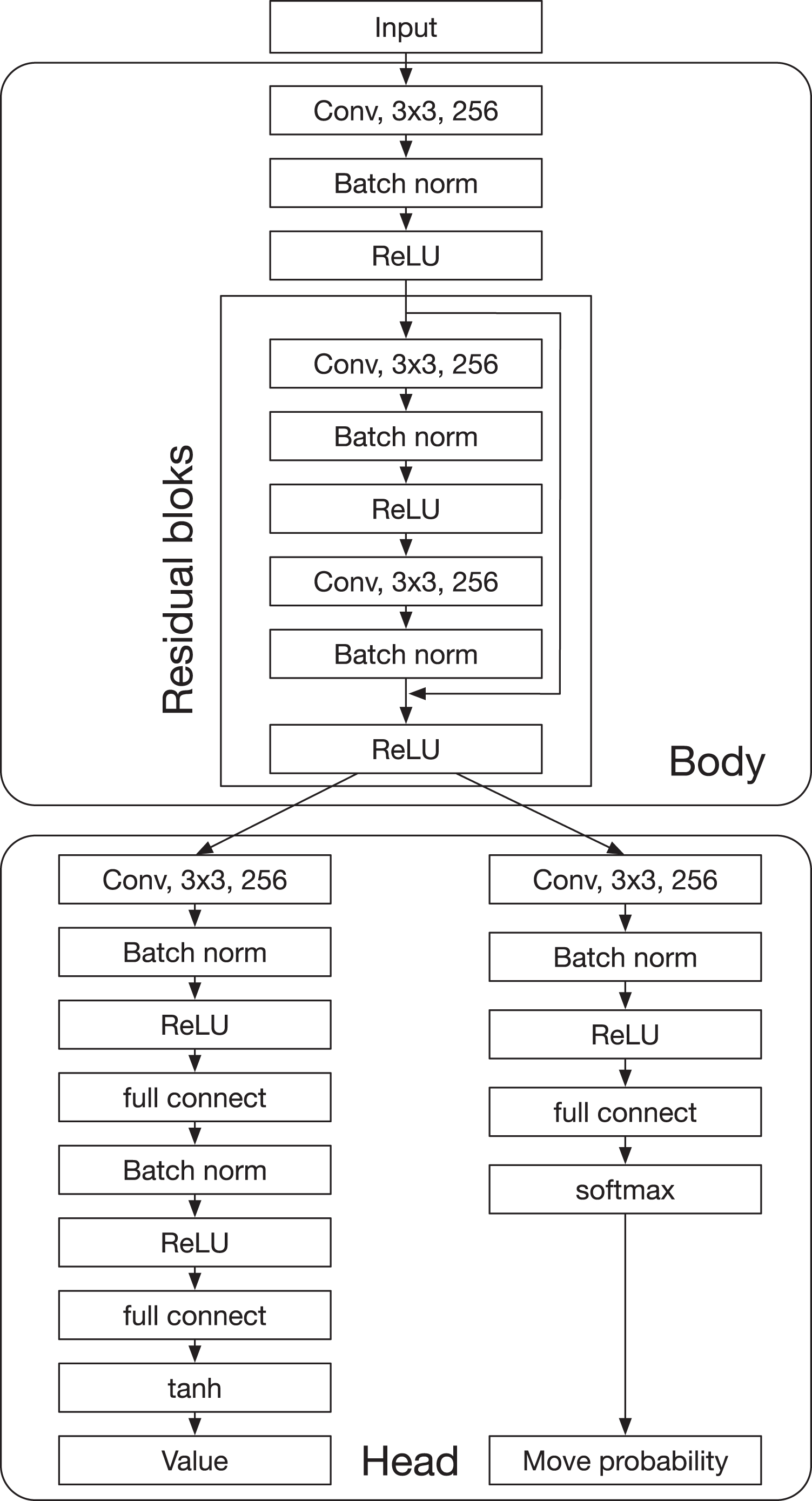
Artificial intelligence (AI) has achieved superhuman performance in board games such as Go, chess, and Othello (Reversi). In other words, the AI system surpasses the level of a strong human expert player in such games. In this context, it is difficult for a human player to enjoy playing the games with the AI. To keep human players entertained and immersed in a game, the AI is required to dynamically balance its skill with that of the human player. To address this issue, we propose AlphaDDA, an AlphaZero-based AI with dynamic difficulty adjustment (DDA). AlphaDDA consists of a deep neural network (DNN) and a Monte Carlo tree search, as in AlphaZero. AlphaDDA learns and plays a game the same way as AlphaZero, but can change its skills. AlphaDDA estimates the value of the game state from only the board state using the DNN. AlphaDDA changes a parameter dominantly controlling its skills according to the estimated value. Consequently, AlphaDDA adjusts its skills according to a game state. AlphaDDA can adjust its skill using only the state of a game without any prior knowledge regarding an opponent. In this study, AlphaDDA plays Connect4, Othello, and 6x6 Othello with other AI agents. Other AI agents are AlphaZero, Monte Carlo tree search, the minimax algorithm, and a random player. This study shows that AlphaDDA can balance its skill with that of the other AI agents, except for a random player. AlphaDDA can weaken itself according to the estimated value. However, AlphaDDA beats the random player because AlphaDDA is stronger than a random player even if AlphaDDA weakens itself to the limit. The DDA ability of AlphaDDA is based on an accurate estimation of the value from the state of a game. We believe that the AlphaDDA approach for DDA can be used for any game AI system if the DNN can accurately estimate the value of the game state and we know a parameter controlling the skills of the AI system.

arxiv-sanity

arxiv-sanity
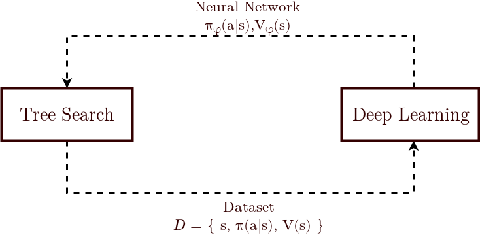
PDF] A0C: Alpha Zero in Continuous Action Space

Figure A1 Deep neural network of AlphaDDA. Full-size DOI
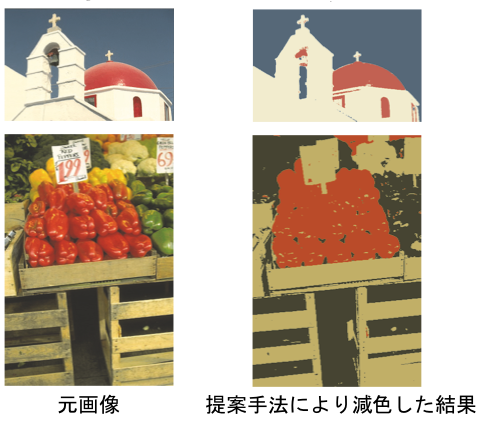
研究概要
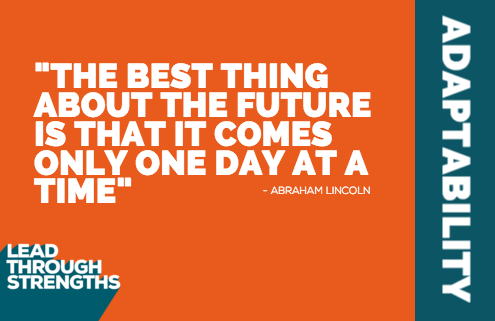
CliftonStrengths Adaptability - StrengthsFinder Talent #3 Of 34

Schematic diagram of the Dynamic Difficulty Adjustment system.

User learning curve Download Scientific Diagram

Games won and lost during the one hundred 30 minute training games. The
Recomendado para você
-
Turnover Chess Variant31 dezembro 2024
-
🔵 AlphaZero Plays Connect 431 dezembro 2024
-
GitHub - petosa/simple-alpha-zero: Clean, tested, & modular31 dezembro 2024
-
GitHub - aqtq314/AlphaZero: A Keras implementation of Google's31 dezembro 2024
-
alpha-zero · GitHub Topics · GitHub31 dezembro 2024
-
redis - golang Package Health Analysis31 dezembro 2024
-
Electronics, Free Full-Text31 dezembro 2024
-
ANN] Announcing AlphaZero.jl - Package Announcements - Julia31 dezembro 2024
-
AlphaZero31 dezembro 2024
-
PDF) Tackling Morpion Solitaire with AlphaZero-likeRanked Reward31 dezembro 2024
você pode gostar
-
Retro-console GIFs - Get the best GIF on GIPHY31 dezembro 2024
-
Sono Bisque Doll wa Koi wo Suru - 02 - Lost in Anime31 dezembro 2024
-
Brinquedo My Little Pony Dia de Princesa Cadance da Hasbro com o Melhor Preço é no Zoom31 dezembro 2024
-
COMO DESENHAR UMA CAPIVARA31 dezembro 2024
-
How the Jazz really lost Gordon Hayward in 201431 dezembro 2024
-
Is Chess Good for Your Brain?31 dezembro 2024
-
File:FNAF World Redacted Banner.webp - EverybodyWiki Bios & Wiki31 dezembro 2024
-
Strike the Blood – TV Series Collection[Blu-ray] - DVD Wholesale31 dezembro 2024
-
Assistir Honzuki no Gekokujou: Shisho ni Naru Tame ni wa Shudan wo Erandeiraremasen Todos os episódios online.31 dezembro 2024
-
Suporte Para Volante Cockpit Simulador SPRO Logitech G27,G29,G92031 dezembro 2024