A survey on heterogeneous transfer learning
Por um escritor misterioso
Last updated 10 novembro 2024
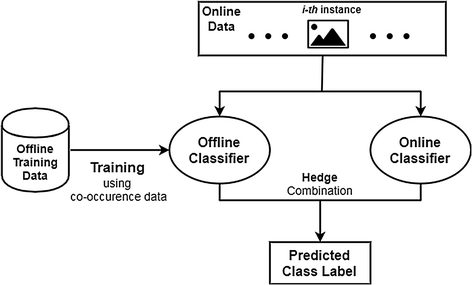
Transfer learning has been demonstrated to be effective for many real-world applications as it exploits knowledge present in labeled training data from a source domain to enhance a model’s performance in a target domain, which has little or no labeled target training data. Utilizing a labeled source, or auxiliary, domain for aiding a target task can greatly reduce the cost and effort of collecting sufficient training labels to create an effective model in the new target distribution. Currently, most transfer learning methods assume the source and target domains consist of the same feature spaces which greatly limits their applications. This is because it may be difficult to collect auxiliary labeled source domain data that shares the same feature space as the target domain. Recently, heterogeneous transfer learning methods have been developed to address such limitations. This, in effect, expands the application of transfer learning to many other real-world tasks such as cross-language text categorization, text-to-image classification, and many others. Heterogeneous transfer learning is characterized by the source and target domains having differing feature spaces, but may also be combined with other issues such as differing data distributions and label spaces. These can present significant challenges, as one must develop a method to bridge the feature spaces, data distributions, and other gaps which may be present in these cross-domain learning tasks. This paper contributes a comprehensive survey and analysis of current methods designed for performing heterogeneous transfer learning tasks to provide an updated, centralized outlook into current methodologies.

Technologies, Free Full-Text
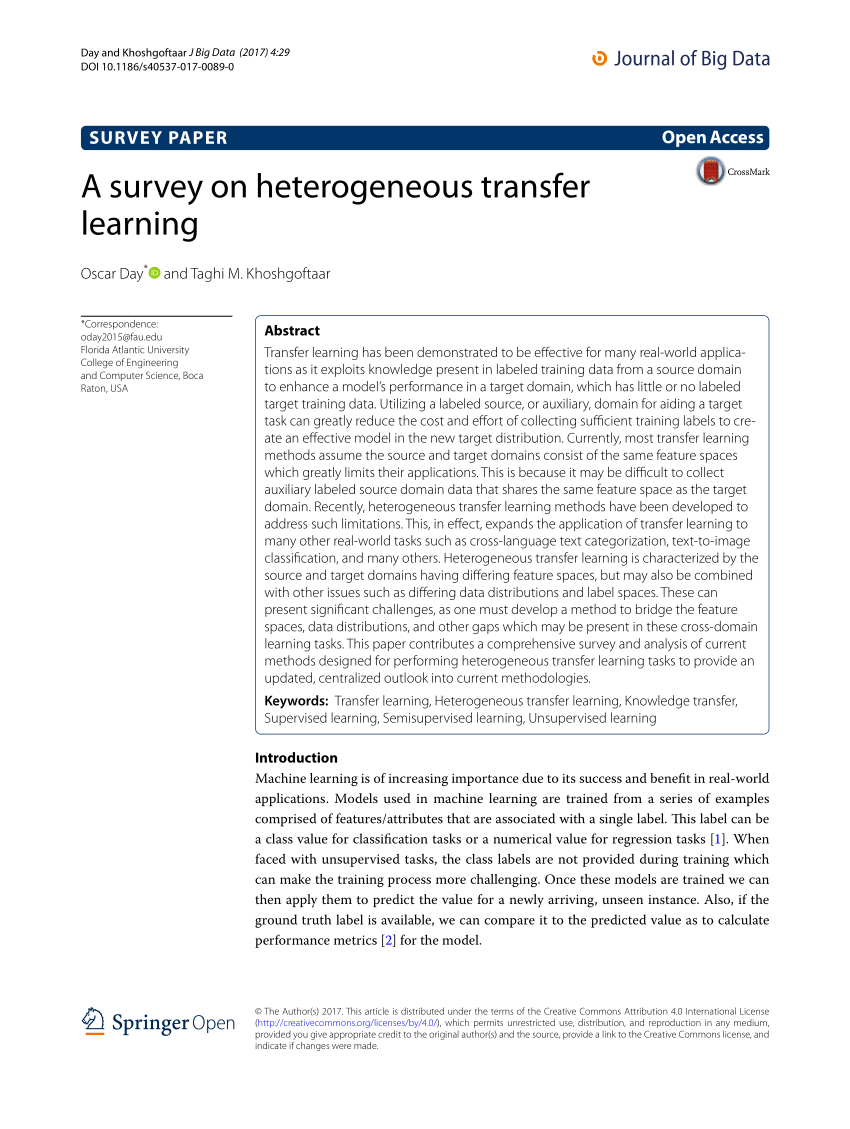
PDF) A survey on heterogeneous transfer learning
Homogeneous vs Heterogeneous transfer learning settings (left

Heterogeneous Federated Learning: State-of-the-art and Research

Evolutionary Reinforcement Learning: A Survey

Recent Advances in Transfer Learning for Cross-Dataset Visual

Transfer learning in demand response: A review of algorithms for

A deep learning framework for Hybrid Heterogeneous Transfer
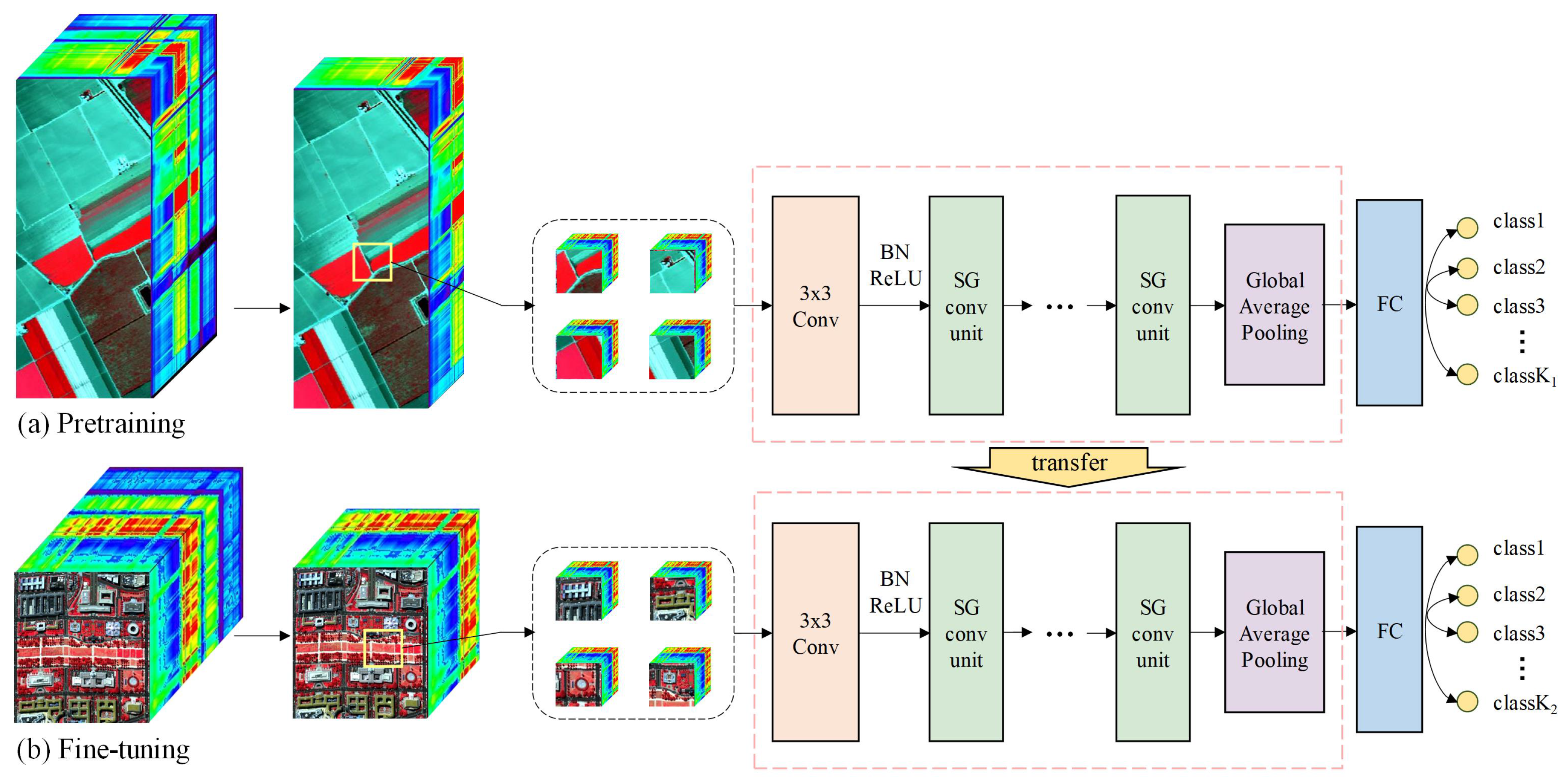
Remote Sensing, Free Full-Text
Recomendado para você
-
Damas Online – Apps no Google Play10 novembro 2024
-
Jogo de Damas :: jogue damas online ou contra o computador10 novembro 2024
-
Baixar Damas - Online & Offline para PC - LDPlayer10 novembro 2024
-
Damas - Online & Offline10 novembro 2024
-
Damas: Online y Offline - Apps en Google Play10 novembro 2024
-
Dames - Checkers Offline Game - Apps on Google Play10 novembro 2024
-
Popn Taisen Puzzle Dama Online Gameplay HD 1080p PS210 novembro 2024
-
Dama - Online & Offline su App Store10 novembro 2024
-
What Is a Data Warehouse Architect?10 novembro 2024
-
Truco Animado10 novembro 2024
você pode gostar
-
FIRE FORCE ONLINE ABILITY TIER LIST10 novembro 2024
-
6 melhores pokémons pra usar em Kanto10 novembro 2024
-
Tensei shitara slime datta ken in 202310 novembro 2024
-
Crossroads - Board Game Online Wiki10 novembro 2024
-
Stranger Things no Behance10 novembro 2024
-
Jogo da velha educativo para imprimir, com três modelos de peças e quatro…10 novembro 2024
-
Providing Managed IT Services In MD, DC And VA >> Fides Tech Solutions10 novembro 2024
-
All Chess Strategy Explained – How to Win in Chess - Remote Chess10 novembro 2024
-
PDF) Realidad aumentada y virtual: valoraciones, percepciones y actitudes del alumnado universitario y su aplicación en el marco educativo.10 novembro 2024
-
Boku no Kokoro no Yabai yatsu Capítulo 118 – Mangás Chan10 novembro 2024